Imagine you’re a developer at Tesla.
You’re working on its Full Self-Driving artificial intelligence (AI) system.
It’s the company’s fully autonomous driving system.
Your job is to feed the AI examples of real-life driving situations for it to learn from.
For most events, that’s not too hard. After all, you’re sitting on 300 million miles of recorded driving.
Need examples of making a right turn at a red light? There are millions of them.
Need to merge onto a highway? Easy.
Now, let’s make it a bit harder.
The U.S. Department of Transportation wants to make sure self-driving Teslas don’t hit a pedestrian dressed in all black who steps out onto the road on a foggy night.
You’d be lucky to find even one example of that on video. Even then, the driver may not have reacted the right way.
Up until recently, this was a problem.
The only solution was to recreate this situation in real life. Tesla engineers had to set up a closed course on a foggy night… and set up a dummy on rails to mimic the actions of a person crossing the road.
Even then, they’d have to recreate it over and over again in different situations – around bends, over hills, at dips in the road.
You see how problematic these rare situations are.
And it’s these rare situations that stand between a degree of autonomous driving where the human driver has to stay engaged… and where they can trust the AI to always make the right maneuver.
Now, researchers have found a way around this problem: give the AI pilot an imagination.
It’s going to be one of the next biggest trends in AI over the next year. And few are talking about it yet.
Imagined Data
The technical term for this AI imagination is “synthetic data.” It’s data made up by one AI to train another.
Let’s look at it through the lens of the same Tesla example.
Developers can use AI to create a video of a person walking out onto the road on a dark, foggy night.
The AI can spit out hundreds of examples or more. Then developers can comb through these clips… pull the best examples… and feed them to the Full Self-Driving AI so it can learn from them.
A problem that could’ve taken months of real-world simulations to overcome could be conquered in less than a week.
This isn’t just an idea either. The AI developer, Wayve, has created GAIA-1 to do exactly what I’m describing.
Here’s a series of AI-generated clips by GAIA-1…
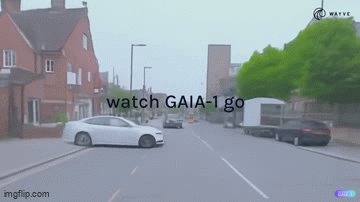
Success with synthetic data has two major applications.
First, it supplements gaps in AI training data. Elon Musk has collected huge amounts of driving data. But even so, there are still rare situations he doesn’t have.
Synthetic data will allow his team of developers to patch these holes quickly.
It’s also a godsend for AI startups that don’t have access to huge amounts of data already like Tesla does.
For months, I’ve been telling you about how Tesla, Meta, Google, Microsoft, Amazon, and other Big Tech companies have a clear advantage in developing AI.
They have access to unparalleled datasets. They also have enough cash to spend billions of dollars on computing hardware to train their AI models.
Synthetic data evens the playing field. Now, a startup, with no data of its own, can use it to train an AI.
Now, a model built entirely on synthetic data won’t be as good as one built on real data. But it’s enough to show a proof of concept.
That can mean the difference between securing funding to get access to more data and computing power or not.
More important, synthetic data holds huge promise for robotics.
Dawn of AI Robots
AI robots present a challenge because most of us aren’t walking around with cameras strapped to our heads.
There isn’t a deep source of data for everyday tasks like chopping an onion or taking out the trash.
In the past, researchers have had to collect this data. This is time-consuming and costly.
But now with synthetic data, they can quickly train these models.
Here’s an example from UniSim. It’s a collaboration between researchers at UC Berkeley, MIT, and Google’s DeepMind AI lab.
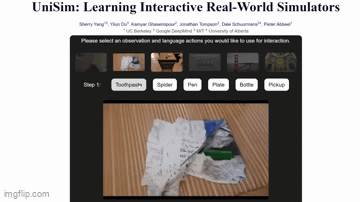
The AI can generate realistic clips of people washing their hands, picking up objects, and even cutting veggies.
Models like GAIA-1 and UniSim are just the beginning of this trend. Developers will refine and expand these models. This will allow them to generate even more realistic clips to train autonomous robots on.
This is why I’m so excited about synthetic data. It’s going to speed up the rate of AI progress. We’re going to see fresh ideas from startups… and we’re finally going to see big breakthroughs in autonomous robot technology.
With synthetic data, developers will be able to build autonomous robots for a wide range of tasks such as washing dishes… folding laundry… and cleaning the floor.
Even better, these robots can learn to operate in new environments. They’ll be able to use synthetic data to imagine scenarios that no developer could come up with… and successfully complete those tasks.
Regards,
Colin Tedards
Editor, The Bleeding Edge
P.S. If you want to learn more about a major AI profit opportunity I’ve got my eye on… Go here to check out my special presentation.
Today, Nvidia is the undisputed king of AI hardware. But as demand for AI chips has skyrocketed – and continues to climb higher yet – even the AI darling has run into a huge problem… It can’t make enough chips.
Which is why I’m not recommending Nvidia today.
There’s a smaller company I call the “next Nvidia.” It will power the next wave of AI profits. And it’s caught the eye of industry giants like Citadel CEO Ken Griffin… hedge fund billionaire David Tepper… and Wall Street legend David Einhorn.
And it’s only a matter of time before everyone starts piling in… because this company is about to unveil the most powerful AI chip in history.